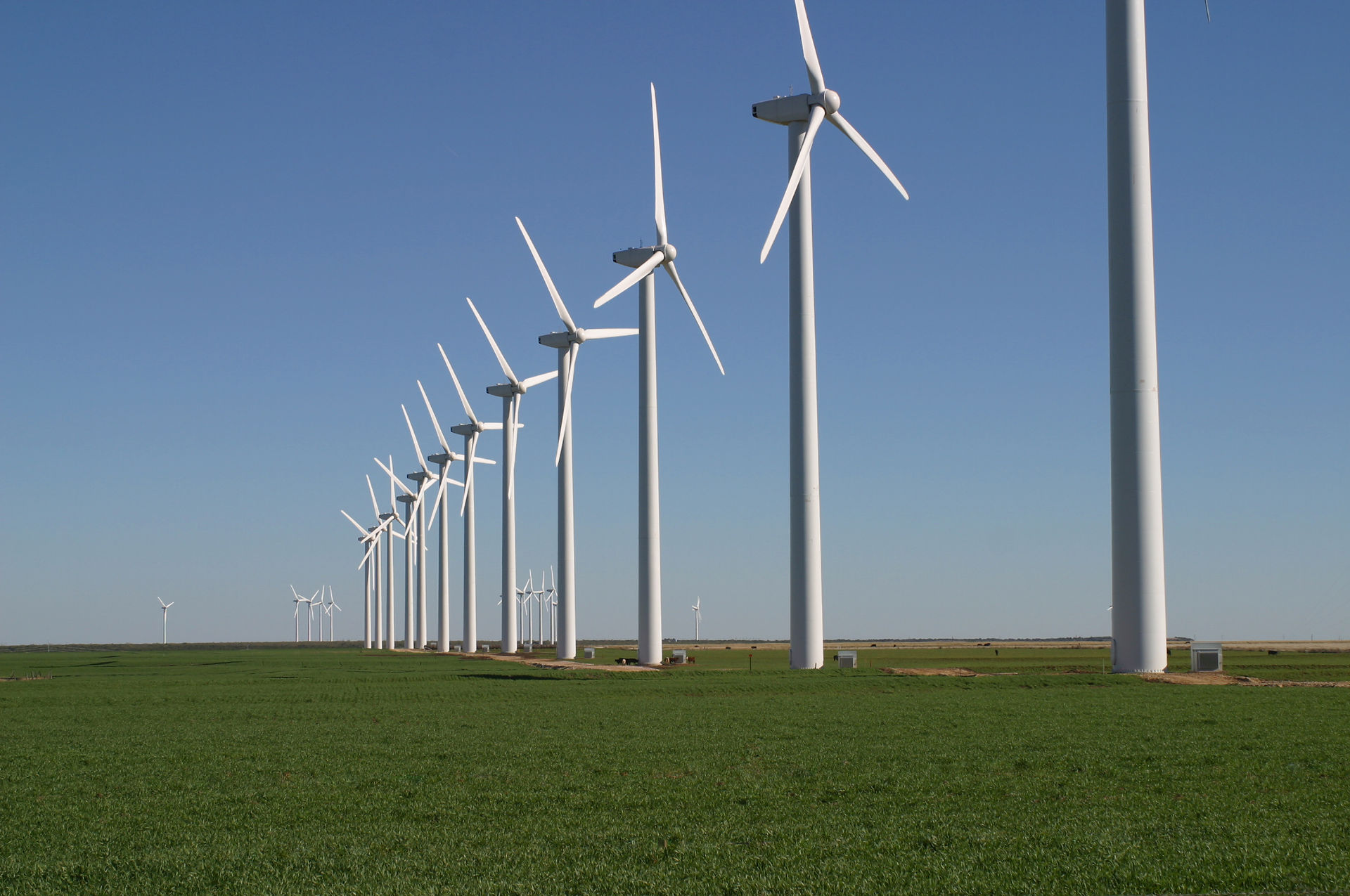
Dissertation
Results and Conclusions
The forecasting system developed for the island of Crete, draws on historical production and meteorological variables (NWP). In fact, the proposed methodology, based on KDE (Kernel Density Estimation), differs from most currently used techniques, for being able to provide not only point forecasts, but also the uncertainty modeling, with different confidence intervals (see example of the figure below).
In order to achieve the final solution, models were created with different features and were analyzed in terms of advantages and behavior , for both types of prediction (deterministic and probabilistic). During this process, it was found that when choosing input variables, the error of only one of these types can be optimized, since the other varies oppositely. In this case, it was considered appropriate to select the model with the best expected production values, adjusting, subsequently, their parameterization in order to optimize the uncertainty's representation. However, this option should take into account what end users want and how forecasts are going to be used. Depending on the situation, it may be more advantageous to target the construction of the model for the probabilistic forecast, from the start.
The study on the parameters to provide the software found that, depending on the system, there are different optimal values. Thus, for each set of data and input variables applied to the training of a model, it will be required a new parameters' optimization, which can give rise to significant improvements in their overall performance.
A small decrease in the forecast error, constitutes a major reduction of the penalties imposed on producers by production variances. Therefore, the quality assurance of the data set becomes crucial. Two methodologies were tested and the one that gave the best results was selected: the average power curve modeling. Also, the use of techniques for the improvement of the forecasts, after its completion, is an important tool in reducing the error, as was the case with dynamic adjustment.
Overall, the final model performed better than the other developed models, and better than other forecasting techniques, such as neuronal networks and the persistence model. The results were very satisfactory for the entire forecasted year , however, as expected, higher errors occur during the winter, due to the considerable variability of the wind resource. One way of improving these results and increase the accuracy of the model is to upgrade, through time, its knowledge base.
Finally, it's relevant to point out the importance of knowing a set of information, compared to only an expected power value, as with the traditional models. The possibility of obtaining the uncertainty associated with each point prediction, allows one to minimize the impacts caused by the volatility of the wind and make the use of wind energy more secure and reliable to all those involved.

Future Work
The reduction of the error between the forecast and the actual power produced will always be a goal in research and development of forecasting systems, however, there will always be some inaccuracies. Therefore, it's important to develop approaches that take advantage of probability forecasts to make decisions based on risk analysis.
The economic benefits of the uncertainty modeling require larger demonstration and proof, because they also have negative aspects that raise questions: additional costs or even complexity of understanding and evaluation. Throughout the development of this dissertation, it became clear the need to create a system that takes into account the preferences of the end users, adapting the model to each case. Also, an improvement to be introduced in KDE software is to integrate heuristic methods capable of automatically optimize the parameterization of the explanatory variables used.
There is also the possibility of developing second-order models, whose input variables incorporate predictions of production variation. However, the prediction of the variation has been found to be very complex, due to the volatile nature of the wind resource, requiring further investigation.
In conclusion, in addition to the implement of improvements in forecasting systems, there is still lot of work to be carried out in developing tools and procedures that make a more efficient use of the information provided by the forecasts of wind generation and it's uncertainty.